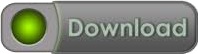
An agent can either be in one state or in the consecutive one, but not in between. However, these means of discretization imply that there is no notion of progress between two consecutive states. These include using linear temporal logic (LTL) formulas ( Calvanese et al., 2002 Pereira et al., 2021), using behaviour trees ( Colledanchise et al., 2019 Sitanskiy et al., 2020) and Fuzzy Finite State Machine (FFSM) models ( Mohmed et al., 2020) among others. Leading approaches to behaviour recognition leverage different means to discretize the states of continuous environments. The identification and characterization of behaviours in complex, real-time continuous environments is a challenging problem which has to account for an unknown sequence of incoming observations and an infinite number of state possibilities. This is owing to the fact that in many instances inter-agent interaction via direct communication could be limited due to faulty devices, network capacity, time limitation, or security concerns ( Heinze et al., 1999 Ilachinski, 2004 Oh et al., 2014). Within the context of a team, behaviour recognition is core to realizing multi-agent coordinated behaviour. Behaviours can be understood as generalized plans, which can be expressed as policies, algorithmic programs, or logical specifications ( Srivastava et al., 2011 Jiménez et al., 2019).īehaviour recognition is a widely researched problem with a wide array of implementations relevant for both adversarial opponent behaviour recognition ( Kabanza et al., 2010) and behaviour recognition within a team of coordinated agents ( Oh et al., 2014). The same behaviour could be executed in different initial conditions and different instances of the environment, generating a range of different sequences of observations. In behaviour recognition we reason about which behaviour explains a sequence of observations, behaviours being more loosely connected with specific domain instances. While plan recognition involves highly complex reasoning to infer which plan best explains a sequence of observations, and goal recognition reasons about which goal best explains a sequence of observations, the problem of behaviour recognition is far more general.

This problem is closely related to plan and/or goal recognition ( Sukthankar et al., 2014). Behavior recognition is the problem of recognizing the behaviour of an agent from an incomplete trace of observations.
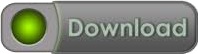